Finding the fault lines
Geographic ML to predict job access in Los Angeles County
CHALLENGE
The uneven spatial distribution of access to jobs that has long characterised American Cities.
PROJECT
Using geospatial machine learning techniques to illustrate that, despite high access to jobs, it is the type and quality of employment that results in the income gap and difference in quality of life for black and white americans in large urban centers.
The uneven spatial distribution of access to jobs that has long characterised American Cities.
PROJECT
Using geospatial machine learning techniques to illustrate that, despite high access to jobs, it is the type and quality of employment that results in the income gap and difference in quality of life for black and white americans in large urban centers.
DETAILS
University of Bristol School of Geographical Sciences + GeoTrans, University of California Santa Barbara, School of Geography
Bachelors in Quantitative Geography thesis
Dr Levi J Wolf and Prof Kostas Goulias, advisors
University of Bristol School of Geographical Sciences + GeoTrans, University of California Santa Barbara, School of Geography
Bachelors in Quantitative Geography thesis
Dr Levi J Wolf and Prof Kostas Goulias, advisors
Finding the Fault Lines explores the inequality of access to employment opportunities in Los Angeles County for different ethnicities.
The uneven spatial distribution of access to employment opportunities has long characterised American cities directly contributing to segregation and income inequality in urban areas. Illustrating this, over 19 percent of Americans living in urban areas are below the poverty level, whereas in suburban locations this decreases to 7.5 percent
The uneven spatial distribution of access to employment opportunities has long characterised American cities directly contributing to segregation and income inequality in urban areas. Illustrating this, over 19 percent of Americans living in urban areas are below the poverty level, whereas in suburban locations this decreases to 7.5 percent
This thesis contextualises the literature on the low upward mobility of the urban poor in American cities. I foreground the multiple definitions and measures of accessibility, and after evaluating recent
attempts to measure accessibility within the framework, I present the need to consolidate approaches to measuring accessibility, whilst incorporating novel statistical techniques that
identify spatial clusters of high and low accessibility. To do so, I develop a machine learning techniques to analyse the level of accessibility to employment opportunities at the block level across Los Angeles County. Furthermore, I compare the results from the standard bayesian modelling technique (multilevel modelling) and the Artificial Neural Network.
I report the study’s limitations and suggest implications for further research.
Together, these methods produce a mode of analysis that attempts to consolidate the methodological approaches to measuring accessibility, to explore the relationship between equity and accessibility for Hispanics and African Americans in Los Angeles County using machine learning techniques.
Together, these methods produce a mode of analysis that attempts to consolidate the methodological approaches to measuring accessibility, to explore the relationship between equity and accessibility for Hispanics and African Americans in Los Angeles County using machine learning techniques.
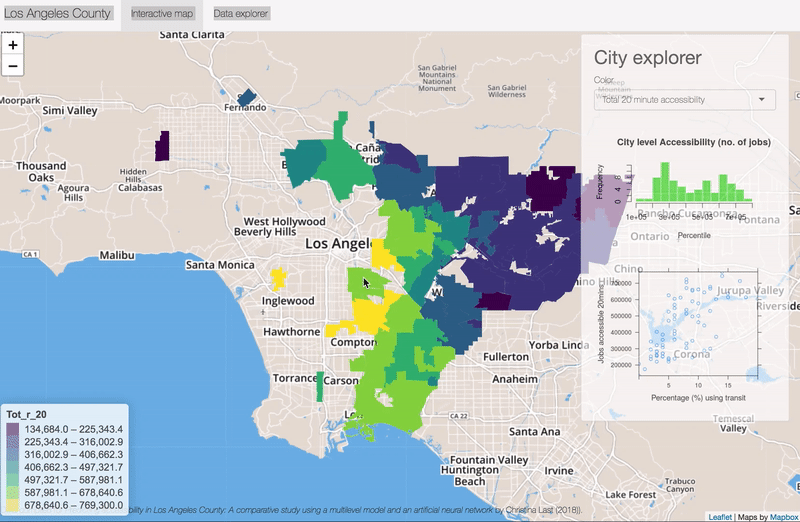
Exposing inequalities in transport planning
The project seeks to highlight the unequal access to employment opportunities for neighbourhoods of high ethnic composition.The aim of the project was to measure the level of influence the geography has on unequal access to jobs in LA. Furthermore, the research questions whether transportation accessibility racially biased? To understand the link between the geography of LA's transportation network, and the socio-economic outcomes of for ethnic communities, I place the machine in the centre of this research question. 'Finding the Fault Lines' is the first comparison of traditional techniques for exploring multiple-scale geographic data (multilevel modelling) to geospatial machine learning techniques to classify accessibility.
Process 1: Methodology
How can geospatial machine learning techniques help our understanding of the racial differences in job accessibility in Los Angeles Country?After mapping the historical underpinnings of the concept of accessibility, I ranked literature on its relevance, based on its applicability, representativeness, and methodological similarity. The methodology devised for the reearch compared a structured approach to modelling multi-scale geography (multi-level modelling) to an unsupervised machine learning technique (artificial neural network).
Process 2: Modelling
How do we measure accessibility?Travel time isochrones spatially identify the locations an individual can reach by transit at certain travel times. Totalling the amount of job availability in the isochrones allows us to understand how many jobs are accessible from any given location in Los Angeles County
Process 3: Prediction
How can new machine learning methods extend the knowledge of transportation accessibility and its effect on ethnic minorities?By comparing the results of a multilevel model which accounts for the different geographical levels accessibility and ethnicity are recorded at, with a supervised machine learning technique, I cross compare the results to evaluate the relative success of each model in predicting accessibility.
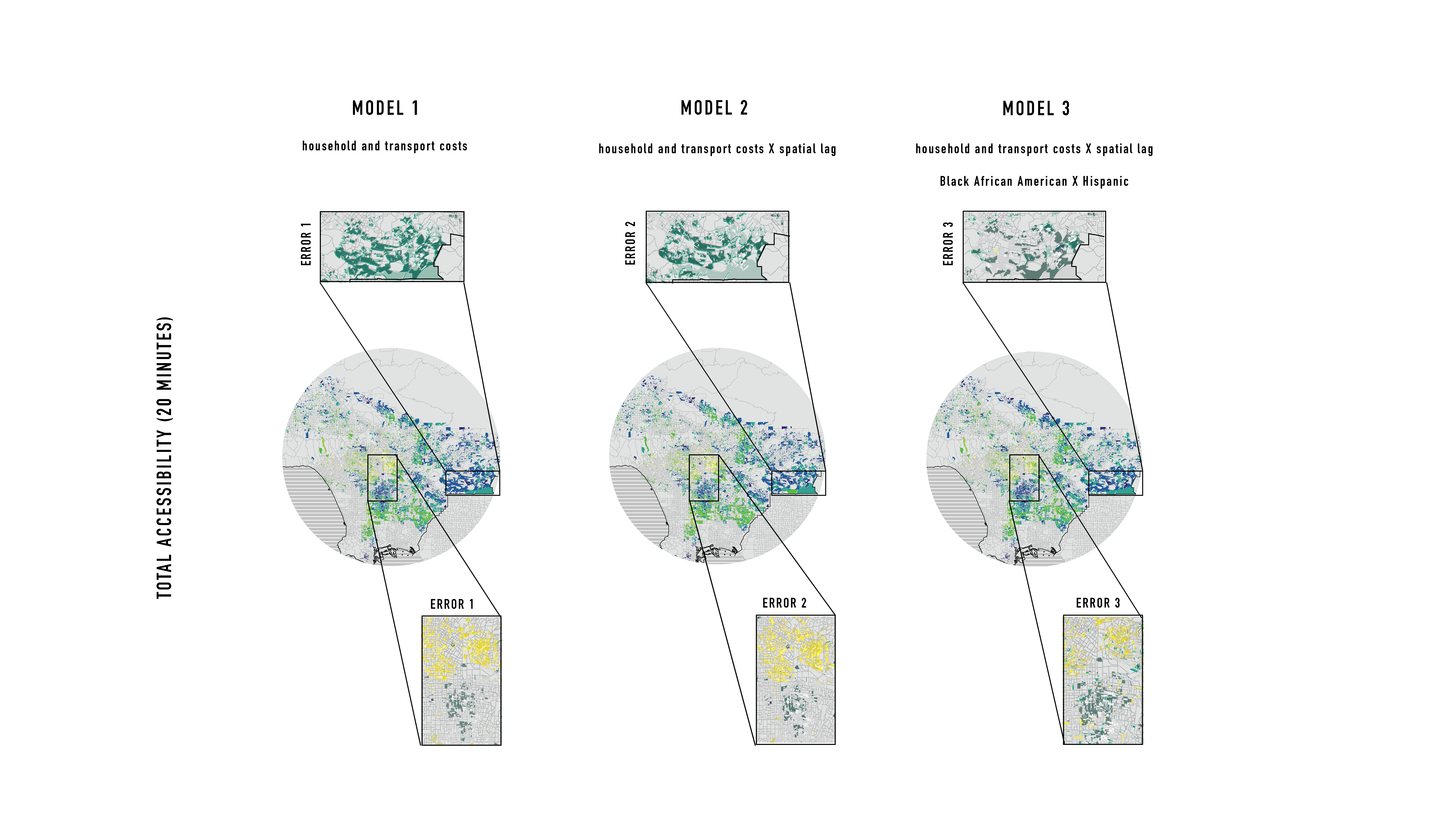
A web interface shows the results of the analysis.
The results were presented at the International Association of Travel Behaviour (IATBR) research conference.
The results were presented at the International Association of Travel Behaviour (IATBR) research conference.